Reliant's paper-scouring AI tackles tedious work in data science
Reliant plans to specialize on the kind of time-consuming, labor-intensive data extraction work that wears out graduate students and interns.
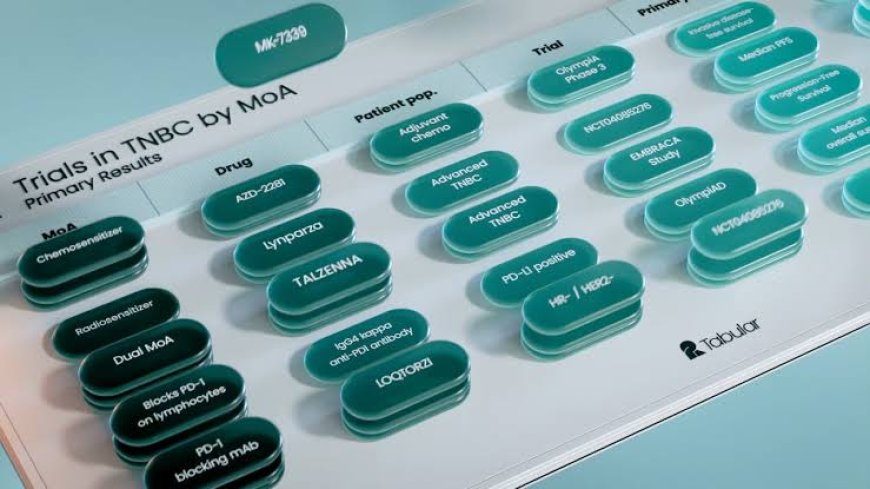
Although AI models have demonstrated a wide range of capabilities, what jobs do we really want them to perform? Ideally tedious work, which is abundant in academics and research. Reliant intends to specialize in the type of labor-intensive data extraction job that currently occupies the time of exhausted interns and graduate students. CEO Karl Moritz stated, "Reducing menial labor and letting people do the things that are important to them is the best thing you can do with AI to improve the human experience." One of the most prevalent types of this "menial labor" in the research field, where he and co-founders Marc Bellemare and Richard Schlegel have worked for years, is the literature review.
Every study references earlier and related research, but it can be challenging to locate these sources in the scientific community. And some employ or quote thousands of data points, such as systematic reviews. Moritz remembered that for one study, "the authors had to review 3,500 scientific publications, many of which turned out to be irrelevant." This felt like something that really ought to be automated by AI because it takes a lot of effort to extract a small quantity of meaningful information.
They were confident that contemporary language models could handle it since, in one experiment, ChatGPT was able to extract data with an error rate of only 11%. It's impressive, like many things that LLMs can accomplish, but it falls short of what people genuinely need.
Tabular, the main product of Reliant, is vastly more successful than an LLM (LLaMa3.1), which it is partially based on. It completed the same task with zero errors, according to the extraction of the thousands of studies mentioned above. This implies that you can throw a thousand documents in and tell Reliant what you want out of them, including this, that, and other data. Reliant then goes through all of the documents and extracts the information, whether it is precisely labeled and organized or—far more likely—not. You may then drill down into specific situations using a beautiful user interface that contains all of the data and any analytics you requested.
Reliant's technology, like any AI application, is highly compute-intensive, which is why the business purchased its own gear instead of renting it on a per-use basis from a major provider. Investing in hardware internally has advantages and disadvantages. Firstly, these costly machines need to be paid for, but secondly, it gives you the opportunity to explore new areas with specialized computing.
Reliant has purchased its own gear instead of renting it on a per-use basis from one of the larger suppliers because, like any AI application, its technology is highly compute-intensive. Bringing hardware in-house has advantages and disadvantages. The cost of these sophisticated devices must be justified, but the opportunity to explore uncharted territory with specialized computing is also present.
What's Your Reaction?
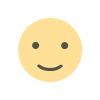
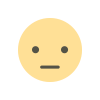
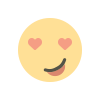
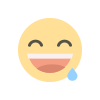
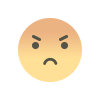
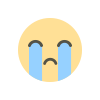
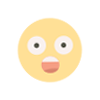